MedAlign
A Clinician-Generated Benchmark Dataset for Instruction Following with Electronic Medical Records
Large language models (LLMs) have demonstrated human-level fluency in following natural language instructions, offering potential to reduce administrative burdens in healthcare. However, evaluating LLMs on real-world clinical tasks remains a challenge. MEDALIGN addresses this by introducing a benchmark dataset of 983 natural language instructions for Electronic Health Record (EHR) data, curated by 15 clinicians across 7 specialties. The dataset includes:
- 302 clinician-written reference responses for instruction-following evaluation.
- 275 longitudinal EHRs to ground instruction-response pairs.
- Comparative analysis of six LLMs, revealing high error rates ranging from 35% (GPT-4) to 68% (MPT-7B-Instruct).
- An 8.3% drop in accuracy when GPT-4 moved from a 32k to a 2k context length.
- Assessment of correlations between clinician rankings and automated metrics, highlighting COMET as the best-performing automated evaluation metric.
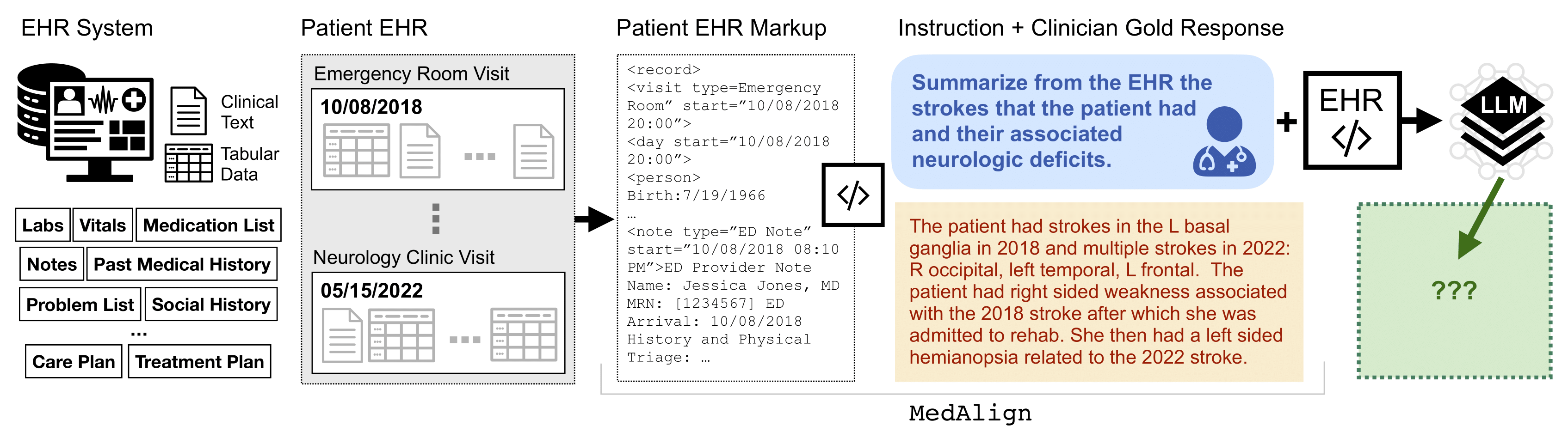